Colbert's fixation
If you are American and have never heard of Stephen Colbert you probably live under a rock. He’s the host of The Late Show, a program I can only describe as “the stand up of news” (plus he also interviews some very famous and interesting guests).
In case you don’t know him already and don’t want to change that, I’ll give you the shortest possible summary of his spiel: he makes fun of Donald Trump.
He’s an outspoken leftist and isn’t afraid to make fun of what he sees as a madman running the White House. Whether you agree with him or not, you’ve got to admit he’s pretty funny…
But even though he’s well known for poking fun at Trump, by watching his show on YouTube almost every day I started getting the impression that maybe he talked a little bit too much about the president. In the end I couldn’t stop myself from asking the question: how much in fact does Stephen Colbert talk about Donald Trump?
Short answer: a lot, like, a lot.
Long answer:
How much exactly?
In my quest to find the answer, the first step was getting the transcriptions of his performances. I created a script that downloaded the captions of about a third of the videos on his YouTube channel (990 to be precise) and decided that was a big enough sample.
Then I had to clean the captions. They were full of symbols (like musical notes), indicators of who was talking and audience actions (clapping, cheering, …) that had to be removed in order for us to be left only with the relevant stuff. Another important step in text mining is getting rid of stop words (stuff like “the”, “a”, “an”, etc.), so I went ahead and did that too.
After that whole process was over, I still had more than 260,000 words of transcribed audio! And even though that’s not 100% Colbert talking, it’s enough of him for us to have a good estimate of what he usually talks about.
The first thing I wanted to know was what words he says the most. Just take a look at the winner…
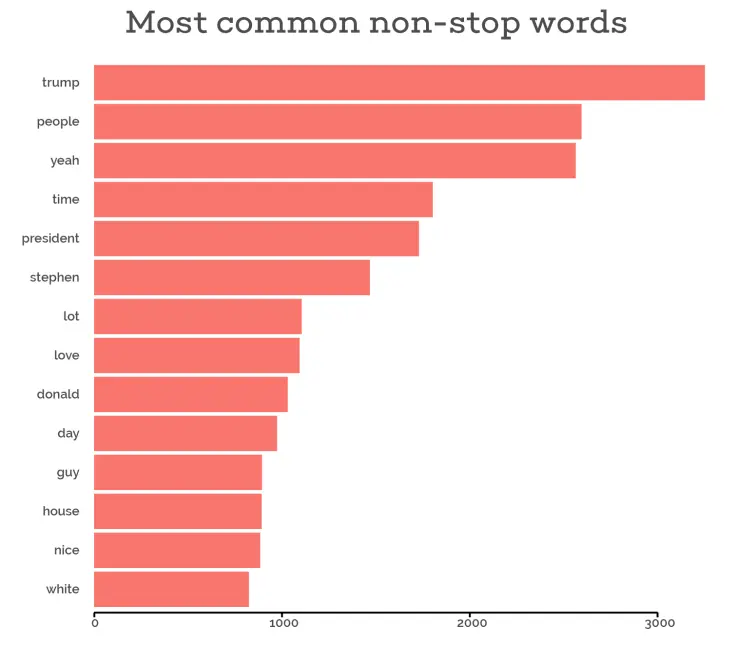
That’s right, excluding stop words, the most uttered word in his show was “Trump”. It came up in the captions precisely 3252 time, making up 1.2% of all non-stop words. If we consider our sample as representative of the 2600+ videos he has on his YouTube channel and if we assume that only half of every episode’s segments end up YouTube and that he’s the one talking for 3/4 of the time, we can conclude that Stephen Colbert has said “Trump” more than 12,000 times on his show!
But just because Colbert says the word “Trump” a lot, it doesn’t necessarily mean that he talks that much about Trump. To find out whether all those 12,000 references are simply out of context utterances I had employ a more complex technique called topic modeling.
No, but really…
According to Wikipedia, a topic model is “a type of statistical model for discovering the abstract ‘topics’ that occur in a collection of documents”. The model I chose is called Latent Dirichlet Allocation (LDA) and I set it up so it would look for 2 topics in our corpus of text.
Just as I expected, the model was able to clearly divide Stephen’s words into two main themes: interviews and politics. We can see that by taking a look at the words the model considered the most characteristic of each topic.
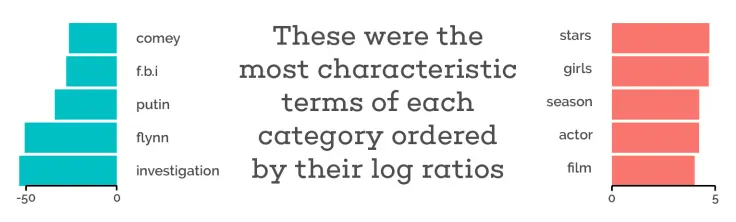
In broad terms, the “log ratio” score on the x-axis of each plot indicates whether a term comes up more often in one topic than another; the more negative the score, the more a term comes up in topic A but not in topic B and vice versa.
Topic A is clearly about politics: other topic A terms not shown in the plot above include “campaign”, “tax” and “republicans”. Topic B on the other hand is representative of Colbert’s conversations with his guests: other terms include “comedy”, “song” and “band”.
But you might be wondering where the T-word went… It doesn’t show up on these plots because there isn’t a topic where it comes up way more often than in the other! With this we can conclude that, not only does Colbert say the word “Trump” a lot, but also that the president is a constant subject of his show even when we only consider his conversations with his guests.
Is that enough Trump for you?
After word
The analysis in this post was made entirely in R. The cleansing and modeling steps were
conducted with the help of the tidytext
and topicmodels
packages. To create the
analytic workflow, I followed Text Mining with R’s examples step by step. My script
downloads all subtitles from ccSubs. And, last but not least, if you want to reproduce the
analysis yourself, the code I used is also available as a Gist.